Table of Contents
- What is Neuromorphic Computing?
- Objective of the Article
- Mini Glossary of Terms
- How Does Neuromorphic Computing Work?
- Real-Life Examples of Neuromorphic Computing
- Benefits of Neuromorphic Computing for Humanity
- Challenges of Neuromorphic Computing
- Statistical Insights into Neuromorphic Computing
- Applications of Neuromorphic Computing Across Fields
- Projections: Neuromorphic Computing in the Future
- The Brain of Tomorrow
What is Neuromorphic Computing?
Imagine a computer that doesn’t just process data like your laptop but thinks and learns like a human brain. That’s the essence of neuromorphic computing. It’s a revolutionary technology designed to mimic the structure and functionality of the human brain, using specialized hardware and software to perform tasks more efficiently and intelligently.
Unlike traditional computers, which process data in a step-by-step sequence, neuromorphic systems work more like neurons in our brain—communicating in parallel, adapting to changes, and solving problems creatively.
Let’s break it down:
- Traditional computers are like workers on an assembly line, performing one task at a time.
- Neuromorphic systems are like a team of musicians in an orchestra, harmonizing to solve complex challenges in real-time.
Objective of the Article
- Explain neuromorphic computing in simple terms.
- Introduce key concepts and terms with a mini glossary.
- Explore its potential benefits for humanity.
Mini Glossary of Terms
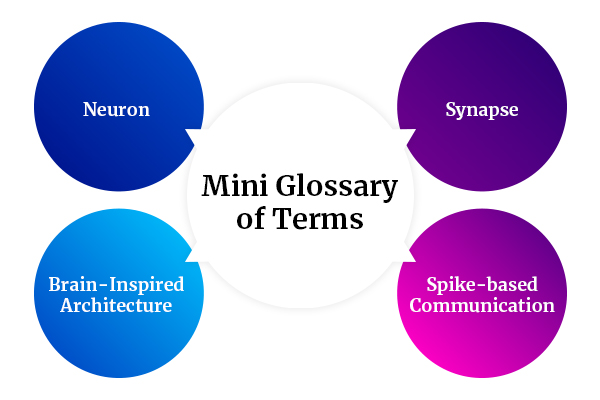
- Neuron: The cells in our brain that process and transmit information. Neuromorphic systems mimic these.
- Synapse: Connections between neurons that allow them to communicate. Neuromorphic chips simulate these to improve learning.
- Spike-based Communication: Just like neurons send spikes of electrical signals, neuromorphic chips use these “spikes” to process data.
- Brain-Inspired Architecture: A design approach for computers that imitates the brain’s network of neurons and synapses.
How Does Neuromorphic Computing Work?
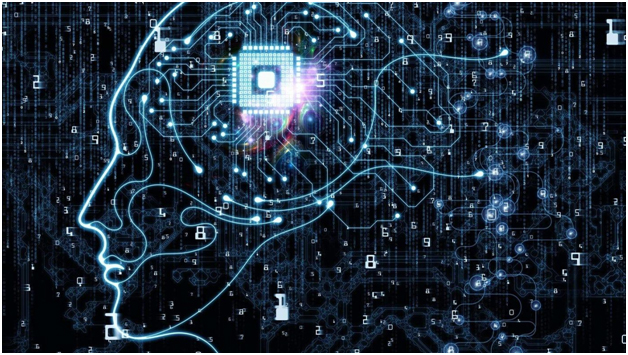
Neuromorphic systems are built using brain-inspired hardware, such as chips that simulate how neurons fire and synapses connect. These systems excel at tasks that are difficult for traditional computers, such as recognizing patterns, adapting to new data, and performing tasks with minimal energy.
For example:
- Traditional computers struggle with tasks like recognizing a face in a crowd.
- Neuromorphic systems process the same task in milliseconds, learning and adapting as they go.
Real-Life Examples of Neuromorphic Computing
1. Intel’s Loihi Chip
Intel has developed the Loihi chip, which mimics 130,000 neurons. It’s used to solve problems like optimizing traffic flow and enabling robotic arms to learn new tasks quickly.
2. IBM’s TrueNorth
IBM’s TrueNorth chip, with over a million simulated neurons, is helping researchers explore AI applications in healthcare and robotics.
3. Brain-Machine Interfaces (BMIs)
Neuromorphic computing enables advanced BMIs that allow paralyzed individuals to control devices using their thoughts.
Benefits of Neuromorphic Computing for Humanity
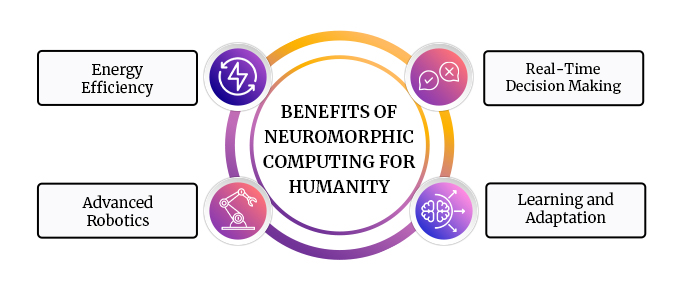
1. Energy Efficiency
- Neuromorphic chips consume up to 1,000 times less energy than traditional computers, making them ideal for sustainable AI applications.
- Stat: Computing energy consumption is predicted to reach 20% of global electricity use by 2030 (IEA, 2023). Neuromorphic systems can significantly reduce this burden.
2. Real-Time Decision Making
- These systems can process and act on data in real-time, making them invaluable for self-driving cars, medical diagnostics, and disaster response.
3. Learning and Adaptation
- Neuromorphic systems don’t just follow programmed rules—they learn and adapt, opening doors to personalized education and healthcare solutions.
4. Advanced Robotics
- Neuromorphic computing is revolutionizing robotics, enabling machines to move, perceive, and interact with the world in human-like ways.
Challenges of Neuromorphic Computing
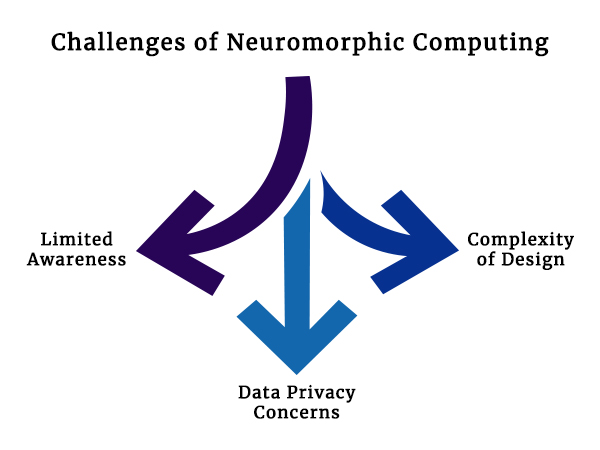
1. Complexity of Design
- Building systems that accurately mimic the brain is no small feat and requires interdisciplinary expertise.
2. Data Privacy Concerns
- Advanced systems that process sensitive data in real-time raise privacy and ethical questions.
3. Limited Awareness
- Despite its potential, neuromorphic computing remains a niche field, with limited understanding and investment.
Statistical Insights into Neuromorphic Computing
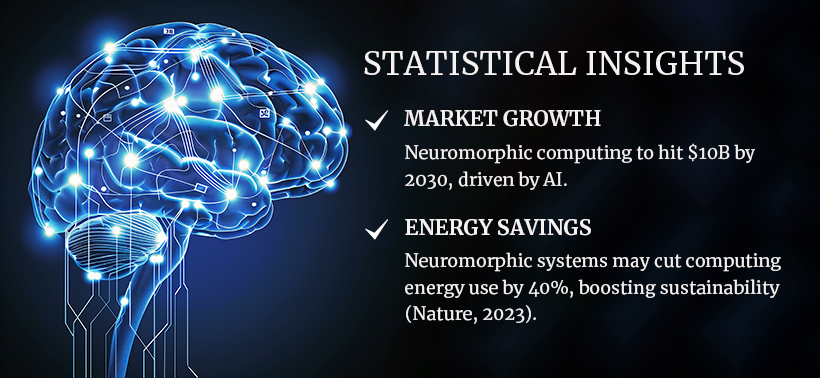
- Market growth: The neuromorphic computing market is expected to grow to $10 billion by 2030, driven by advancements in AI and IoT (Markets and Markets, 2024).
- Energy savings: Neuromorphic systems could reduce global computing energy use by 40%, addressing sustainability challenges (Nature, 2023).
Applications of Neuromorphic Computing Across Fields
1. Healthcare
- Early diagnosis of diseases using pattern recognition.
- Brain-machine interfaces for prosthetics and rehabilitation.
2. Autonomous Vehicles
- Real-time object recognition and decision-making.
3. Smart Cities
- Optimizing energy grids, traffic management, and resource allocation.
4. Education
- Adaptive learning systems tailored to individual students.
Projections: Neuromorphic Computing in the Future
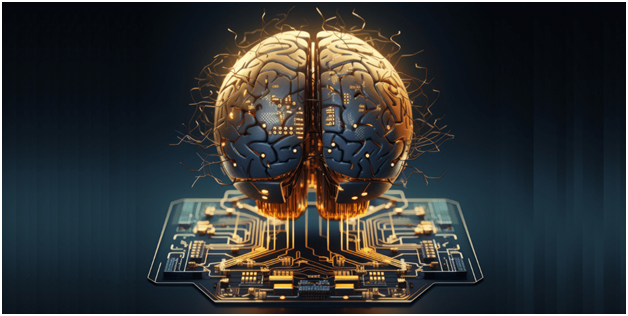
1. Brain-Inspired AI Systems
AI systems powered by neuromorphic chips will blur the line between artificial intelligence and human cognition.
2. Global Energy Savings
Neuromorphic computing will play a critical role in reducing the environmental impact of growing data demands.
3. Human-Machine Collaboration
From healthcare to space exploration, neuromorphic systems will enable seamless collaboration between humans and machines.
The Brain of Tomorrow
Neuromorphic computing represents a giant leap forward in technology, bridging the gap between traditional computers and human cognition. By mimicking the brain’s extraordinary capabilities, these systems offer unparalleled opportunities for real-time learning, energy efficiency, and innovative applications across industries.
As we look ahead, the true potential of neuromorphic computing lies in its ability to redefine what machines can do—not just processing data but thinking, adapting, and collaborating like never before. The future of technology isn’t just smarter; it’s becoming more human.